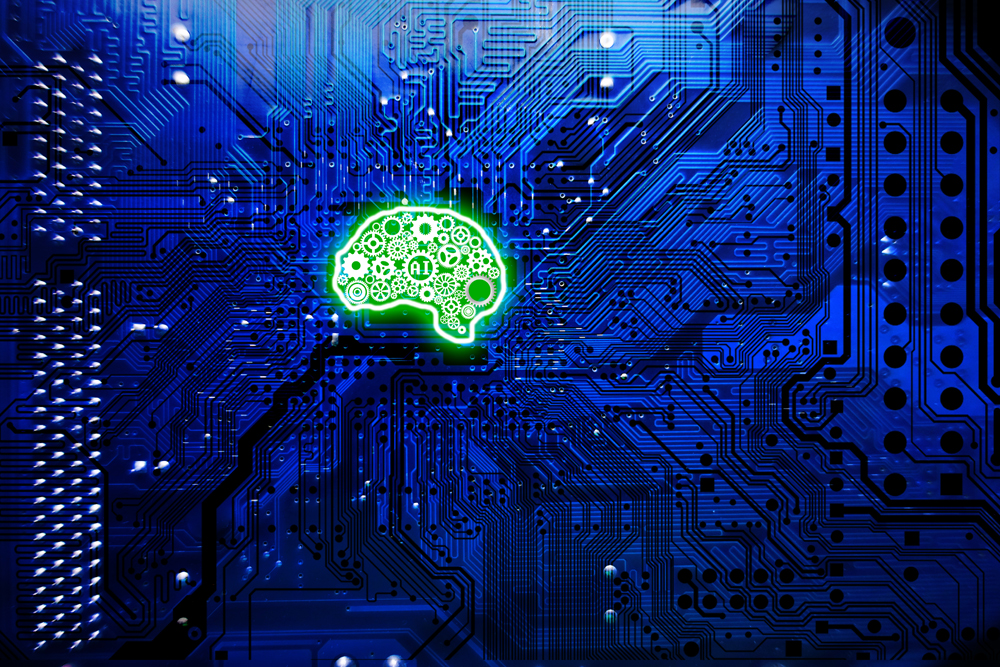
Artificial intelligence (AI) is fast gaining recognition as a key tool for supporting more extensive and less expensive delivery of effective road management and maintenance regimes. It is responding to growing demands for speedier and more budget-conscious solutions to growing highway structural issues and traffic pressures.
The American Society of Civil Engineers (ASCE) recently published its 2021 Report Card for America’s Infrastructure, which concludes that over 40% of the country’s 6.5 million km public highway network is currently in “poor or mediocre” condition - a number that has remained stagnant over the past several years.
This is resulting in users having to pay out nearly $130bn per year on wasted time and fuel - and repairs. One new industry response comes from RoadBotics, a company spun out of Pittsburgh’s Carnegie Mellon University Robotics Institute in 2016 to specialise in developing AI and machine learning for road condition assessment and repair prioritisation.
GIS-based platform
Its GIS-based RoadWay platform analyses given stretches of highway in 3.3m sections to generate the reliable condition ratings and imagery needed to support cases for remedial work in local budgeting and decision making regimes.The previous approach being adopted by one client, the city of Savannah in Georgia, included the recruitment, training, equipping and supervision of employees at a total cost of some $130,000 over three years – this appearing to be the best cost option available at the time (see table below). But its relative slowness meant covering only one-third of the city’s entire 1,120km road network each year, and obliging city officials to use outdated information when it came to budgeting and forward planning. The company’s delivery of an AI-based alternative has saved the city over $80,000 to date, which has been directed to boost spending on proactive maintenance. Savannah has also received shapefiles of the assessment data generated, for future reference, and these have proved compatible with its existing in-house GIS system.
Again in North America, the Eye for Infrastructure spin-off from Canada’s University of Waterloo is currently field-testing automated AI software that automatically analyses vehicle-mounted mobile phone or dash cameras on dedicated municipal trucks and similar vehicles to identify pavement defects. Engineering Professor John Zelek sees this as enabling more timely and lower-cost repairs, resulting in lower taxes for residents, while offering results that are “at least comparable” with those gained by conventional means such as visual inspections.
The project began by using online images from Google Street View, but negotiations showed that this could cost a small community of, say, 30,000 people over $82,000 annually for images that might not be refreshed as frequently. The company is also applying the AI software to images from other sources, including a specialist company that has developed a partially-automated system for detecting pavement defects. The aim overall is to deliver consistently lower taxes for community residents.
Automated data analysis
Meanwhile, the National Research Council (NRC) of Canada has delivery of smart and sustainable pavement structures as a key aim under its Scale AI programme. One $2.6m NRC project aims to boost nationwide supply chain performance through the better management of “uncertainties and variability” in freight transport.
In Europe, Vaisala is claiming credit for a series of cost- and time-saving user benefits now being delivered by enhanced versions of its RoadAI technology. This allows data to be collected on the same journey as a routinely-scheduled safety inspection or regular road patrol. With low-cost equipment (basically a camera or a smartphone) and automated data analysis, the Finnish company claims that there is no need for trained inspectors; while information can be gathered while using any vehicle.
The technology is also designed to carry out defect inventories at normal highway speeds, so minimising the risk of traffic disruption. It aims to deliver output within fewer than 48 hours of a survey run, compared with historic instances of weeks; with one local government reporting that it can survey “almost anything it wants” every six months using five smartphones.
In a further enhancement, the system has adapted in response to indications from early users that they wanted to be able to make real-time comments alongside their captured video footage while en route, to enhance the content and quality of their records. The company has dealt with the issue by introducing a hands-free spoken note functionality, the output from which can be exported as text files for task-sharing. By using the system, it reports, with Vaisala RoadAI installed on just five smartphones, an individual local government can survey “almost anything it wants” every six months. The system also claims to eliminate subjective interpretations arising from “coarse visual” inspections.
Satellite image capture
Encouragingly for the scope for widescale take-up, benefits are becoming increasingly evident for smaller, local, as well as large-scale, highway networks. The UK’s Blackpool town government has so far saved over £1 million in filling in over 5,000 potholes using AI to detect, in seconds, road and (as importantly) road markings damage using space satellite image-capturing technology from condition survey specialist Gaist. Poorly maintained markings are increasingly being seen as a key safety issue, since they can make it difficult for motorists to know for certain where they can appropriately park, overtake or change lanes.
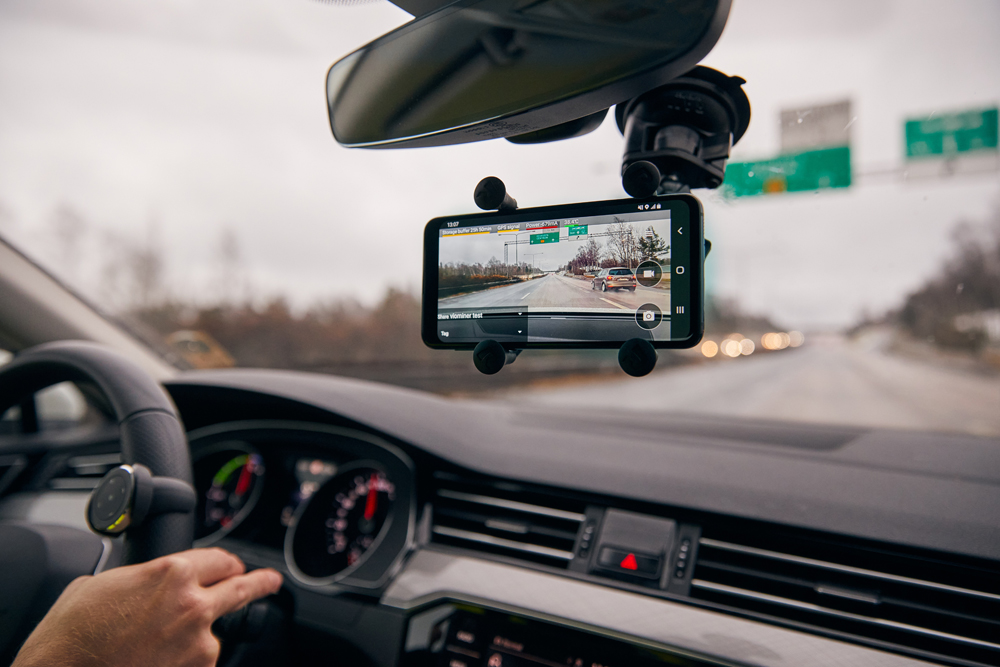
The approach is saving on traditional methods commonly used by lower-tier communities, which tend to involve the physical despatch of individual inspectors (whose travel-to-site times also have to be taken into account). A parallel intervention, again by Gaist has cut the community’s road-related vehicle damage claims from £1.8m a year to under £100,000. The scheme forms part of a UK Department for Transport (DfT) £2m initiative that is exploring the scope for a national scheme deploying AI and machine learning on highways.
Working with the country’s recently-formed national Local Council Roads Innovation Group (LCRIG), it aims to help local governments assess where best to allocate their planned investments in highway networks. DfT also intends in future to support major surveys of footpaths and cycle routes, which currently have low maintenance priorities, again using AI.
Its head of local roads, Steve Berry, says: “We hope the approach will act as a showcase of what can be achieved by local governments working in partnership with the supply chain”.
Warning shot
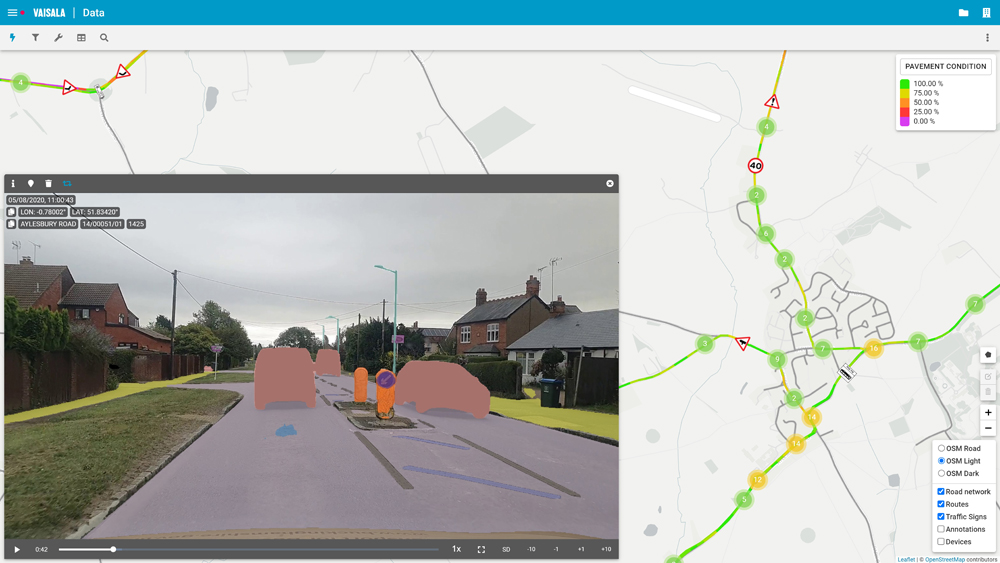
But a good deal of work remains to be done in ensuring that local governments can consistently exploit the full value-for-money potential of the technology, according to Gaist chief technology officer Dr Stephen Remde. In an article published by LCRIG, he warns that AI as currently deployed “has a limited awareness of context, which is important as the location and surroundings can make a defect more or less of a risk”.
He points out that in highways surveying, the work that AI is doing simply categorises objects and features – and that this is not ‘intelligent’. “There is a long way to go before AI can understand the full implications and remedial actions necessary or prioritise actions based on risk,” he concludes. “We are not at the point yet where we can just leave it make decisions for us – especially, for example, in the context of safety on the highway network”.
ABOUT THE AUTHOR:
David Crawford has spent decades writing about and researching ITS and is a contributing editor to ITS International