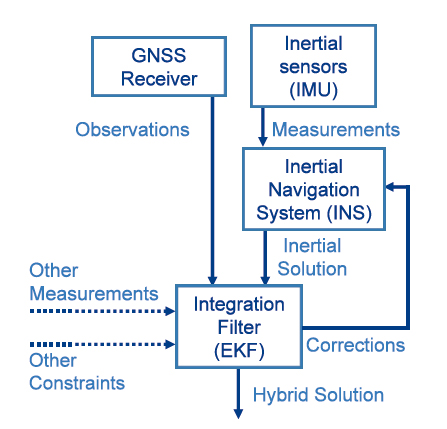
Phil Harris,
Global Navigation Satellite System (GNSS)-based positioning is unique among available positioning technology due to its universal coverage and low equipment cost. By measuring the distances between an unknown position (such as a vehicle), and at least three known positions (GPS satellites), the unknown position can be calculated in three dimensions (latitude, longitude, and height). This is known as trilateration.
However, to resolve a fourth dimension - time - GNSS actually requires measurements to at least four satellites.
For many ITS applications the use of a GNSS-only solution has significant disadvantages, primarily caused by the extremely low power of the received GNSS signals. This low power makes the signals vulnerable to interference (either intentional or accidental) and poor availability due to physical obstruction.
Signal reflections and blockage are particularly prevalent in built-up urban environments. Furthermore the proliferation of low-cost GPS jammers (or 'personal protection devices' as they are known in the USA) is causing increased levels of concern across many user communities. Such threats make the performance of the positioning system of critical concern for liability-critical systems such as Road User Charging (RUC).
Despite its limitations GNSS remains a highly desirable source of position information. GNSS receivers can provide two major types of data: solution data which gives the absolute position, velocity and time of the user; and observation data which gives the distance and relative speed between the user and each satellite in view. In most applications the solution data is used directly, with observation data typically being used for scientific or other high-precision applications. However to produce a single set of solution data, observation data must be available for at least four satellites - when visibility is restricted (such as in urban environments) the inability of the receiver to 'see' at least four satellites will result in solution data being unavailable. Furthermore, because the GNSS receiver is effectively receiving all of the satellite signals at a discrete point (the antenna), it is not possible to determine the orientation of the platform on which the receiver is mounted.
These limitations can be largely resolved by augmenting GNSS data with additional measurements relating to the motion of the vehicle, particularly those that can be obtained without reference to external systems. By accumulating these measurements relative to a known initialisation point it is possible to 'bridge' the periods when GNSS-derived positions are unavailable, or to improve the confidence in the position estimate when GNSS is available.
Inertial sensors
Inertial sensors measure the motion of the platform to which they are attached without reference to an external system.
Full measurement of motion requires a pair of sensors for each of the three axes (forwards, right and down). Typically each pair consists of an accelerometer for measuring linear acceleration along the axis and a rate gyro for measuring the rate of rotation around the axis. By integrating these six measurements it is possible to recreate the trajectory of the platform relative to an initial position and attitude. These sensors and the associated mathematical algorithm constitute an Inertial Navigation System (INS).
In recent years the rise of motion-sensitive consumer devices such as mobile phones and games consoles has dramatically reduced the cost of the required sensing devices to a total of a few dollars, a level where the incremental cost of including them in typical ITS equipment is negligible compared to other system components.
The major weakness of inertial sensors is that they only measure relative motion and therefore the INS requires initialisation to a known absolute position. Furthermore the outputs of inertial sensors often include considerable bias due to the limits of manufacturing, and this can cause significant apparent positional and rotational drift in the output of the INS unless periodic corrections can be applied by an external absolute positioning system. However the solution generated by the INS is always available, independent of any external system, and the INS can also provide an estimate of the attitude (true heading, roll, and pitch) of the platform.
Given the complementary attributes of GNSS and inertial sensors it is therefore attractive to combine both devices to generate a single highly available solution: the GNSS receiver providing absolute position and velocity data but with dubious availability, while the INS provides continuous position, velocity, acceleration and attitude data once it has been initialised.
Sensor fusion
Figure 1 (the tightly coupled sensor fusion architecture) combines measurements from the GNSS receiver and INS, and potentially measurements from other sensors, into a single composite position estimate via the Integration Filter. The Integration Filter is an Extended Kalman Filter (EKF), a mathematical algorithm which as well as generating the Hybrid Solution also estimates the factors applied to the INS to correct errors in the inertial sensors.
In normal operation the output of the Integration Filter is dominated by the accuracy of the GNSS Solution, although the INS does effect some filtering to reduce noise. The accuracy to which the Hybrid Solution can be maintained in the absence of GNSS is largely determined by how well the Integration Filter could estimate and therefore correct the bias terms in the inertial sensors before GNSS data was lost, and the long-term stability (quality) of the inertial sensors.
The range and range rate observations provided by the GNSS receiver to the Integration Filter represent the geometric distance between the vehicle and each satellite, and have been corrected for atmospheric propagation effects and other error sources. The receiver also provides data that allows the Integration Filter to calculate the position of each satellite for any instant, allowing the filter to convert the range measurement into a 3D vector between the known satellite and platform positions.
These individual GNSS measurements act as 3D constraints on possible vehicle motion, allowing the Integration Filter to continue stabilising to the INS even when only a single satellite is visible, providing higher accuracy during extended periods of limited sky visibility (although at least four satellites are still required for system initialisation). This is a significant advantage over the more common approach of position-domain sensor fusion (the 'loosely coupled' architecture) that requires a full position solution (four satellites) from the GNSS receiver to obtain any benefit from GNSS.
Figure 2 presents an example of the performance achieved during vehicle trials with a consumer grade GPS (Global Positioning System) receiver and typical inertial sensors. The green points are the standalone GPS position fixes. The combined solution is represented by the yellow line, with its associated ±1Û (±34 per cent) error estimates as red lines.
Figure 3 shows an example of the performance achieved during vehicle trials when using the closely coupled architecture with a professional-grade GPS receiver and the same inertial sensors. In this example the green points representing the standalone GPS position fixes show very poor availability in the urban environment, including outages in excess of 60 seconds. In contrast, the combined solution (yellow) line and its associated 1Û (single-sided) error estimate (red line) accurately reconstruct the route taken by the vehicle.
Although full position fixes are rarely available from the GPS receiver, the individual range measurements are sufficient to constrain the INS and therefore the estimated accuracy of the combined solution over extended periods of time. In this example the position error is typically maintained to within 3.3m after any 30 second outage.
Summary
The performance of most navigation systems can be expressed in the following terms: accuracy (How well do I know my position?); continuity (Will I have continuous solutions over the next n seconds?); integrity (What confidence can I have in my position?); and availability (Can I get a position here? Right now?).Continuity and availability are 100 per cent when inertial aiding is used - once the system is initialised a position solution is always available. Accuracy and integrity are primarily determined by characterisation of the inertial sensors in terms of biases and noise, and the number of good GNSS measurements that have been received to enable this. Accuracy is improved through the inherent averaging behaviour of the Integration Filter as it effectively compensates the GNSS measurements for any motion effects. The availability of inertial data allows a robust independent estimate of the achieved accuracy and the integrity of the GNSS measurements, allowing standalone detection of spoofing or other attacks on the installed equipment.
In urban environments, navigation systems using only GNSS fail to meet any of the four key positioning metrics at a level sufficient for liability-critical ITS applications. In many cases the achievable performance cannot even be determined. However, as demonstrated here, the inclusion of inertial aiding can address these deficiencies and dramatically improve both accuracy and overall system reliability in the presence of such difficult environments.