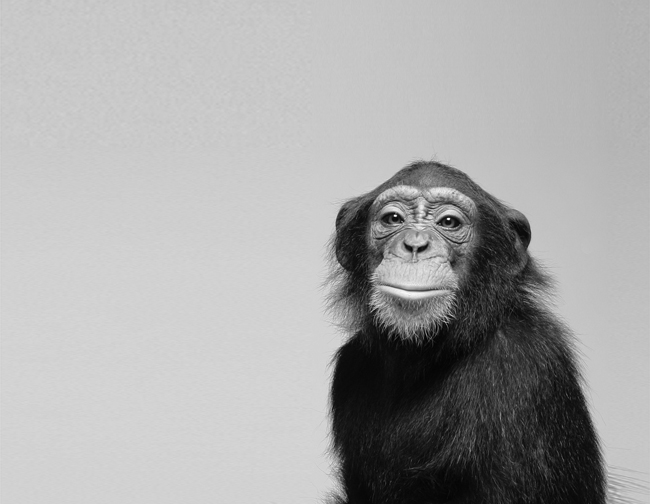
Pointing at objects – be it with language, using gaze, gestures or eyes only – is a very human ability. However, recent advances in technology have enabled smart, multimodal assistants - including those found in cars - to action similar pointing capabilities and replicate these human qualities. Through the use of image recognition and deep learning, smart assistants are revolutionising autonomous vehicles and showing us a future in which our cars are going to be able to point at - and define - objects.
As we learn more about the world around us, we’re finding that there are few things that only humans can do. How about counting? Birds can deal with numbers up to 12. Using tools? Dolphins are using sponges as a tool for hunting.
It may come as a surprise that the animal kingdom can do these tasks. But it highlights how unusual pointing is in being specific to humankind. While it seems natural and easy to us, not even a chimpanzee - our closest living relative - can do more than point to food that is out of its reach in order for humans to help retrieve it for them. Interestingly, this only happens in captivity, suggesting they’re copying human behaviour and they don’t understand when a human helper points to a place where food is hidden, despite a young child understanding this quite easily. So, how can we possibly expect machines to understand it?
The term ‘multimodal’ is often positioned as providing the choice between modalities, for example, typing - or speaking - or handwriting - on a pad to enter the destination into your navigation system, but it’s important to remember that this is not true.
In reality, multiple modalities should work together to accomplish a task. For example, when pointing to something in the area (modality 1) and saying, “tell me more about this” (modality 2), both speech and gaze recognition is needed to explain what the user wants to accomplish. Imagine being in your car, driving down the high street and wanting to find out more about a restaurant that appeals to you; there is the possibility to look at it and simply ask “tell me more about it”, thereby using both modality 1 and 2.
Human-like response
As the technology develops, it’s hoped that more information will be available to the systems: for example, a driver may be able to find out whether there is free parking at the restaurant in question or what vegetarian options there are on the menu.
Being able to point in the visible vicinity is now also available in smart auto assistants. Earlier this year, at CES (formerly known as Consumer Electronics Show) in Las Vegas, Nuance introduced new Dragon Drive features to show how drivers can point to buildings outside the car and ask questions like: “What are the opening hours of that shop?” in order to engage the assistant.
Perhaps, what is more amazing is that the ‘pointing’ doesn’t need to be done with a finger (something which is vital when a driver’s hand should remain on the wheel). This new technology enables users to simply look at the object in question, made possible by eye gaze detection, which is based on a camera tracking the eyes, combined with conversational artificial intelligence. The assistant can then resolve the point of interest and provide a meaningful, human-like response.
For many years, biologists have explored gaze detection in humans and suggested the distinct shape and appearance of the human eye (a dark iris and a contrasting white surround) enables us to guess where somebody is looking, just by observing their eyes. Artists too have examined this phenomenon; with just a few brush strokes they can make figures in their paintings look at other figures or even outside the picture – including the person viewing the painting. For example, in Raphael’s Sistine Madonna, the figures are painted to ensure they point at each other, which in turn guides our view.
Now machines are beginning to have the capability to do this, using image recognition based on deep learning. These skills will take us into the age of true multimodal assistants.
Possibilities are endless
While this technology is in the early stages of development, its potential is not only limited to the automotive industry, but also in the wider transportation sector to assist with urban mobility.
In the future, cars will sense when dynamic and static objects (such as buildings) are using the available real-time map data and will be able to navigate the passenger to their destination via the quickest possible route.
While these capabilities are clearly hugely attractive to today’s drivers, there are clues that it might be even more important as autonomous vehicles become the norm. Many people are beginning to wonder what drivers will do when they don’t have to drive anymore, and become passengers – something they would experience in Levels 4 and 5 of the autonomous driving scale. A recent study found that, if alone, the top activity would be to listen to the radio (63%), while with a co-passenger drivers would be most interested in having a conversation (71%).
It is therefore not too difficult to imagine a future of gaze and gesture detection, combined with a ‘just talk’ mode of speech recognition, that lets users engage the virtual assistant without having to say any start phrase, such as “OK Google”. And for today’s users of truly multimodal systems, machines just got a little more human-like again.
Three forms of pointing
Scientists believe they have found the cause of why pointing is easy for humans but less so for apes. It’s all linked to human language. In 1934, the linguist and psychologist Karl Bühler offered three forms of pointing, all of which connected to language. The first is demonstration (or ‘ad oculos’), which is in the field of visibility centred around the speaker (‘here’), but also accessible to the listener. While it’s possible to point within this field with just our fingers, language can offer a special set of pointing words that complement this action, for example: ‘here’ versus ‘there’, ‘this’ versus ‘that’, ‘left’ versus ‘right’, ‘before’ and ‘behind’, et cetera.
The second form is similar, but it operates in a remembered or imagined world, brought on by the language of the speaker and listener - for example: “When you leave the Metropolitan Museum, then Central Park is behind you and the Guggenheim Museum is to your left. We will meet in front of that”.
Finally, the third form is pointing within language. As speech is embedded in time, we often need to point back to something we said earlier or point forward to what we will say in the future.
This anaphoric use of pointing words - such as: “How is the weather in Tokyo?” “Nice and sunny.” “Are there any good hotels there?” - can be supported in smart assistants (although these capabilities can distinguish the smart from the not-so-smart).