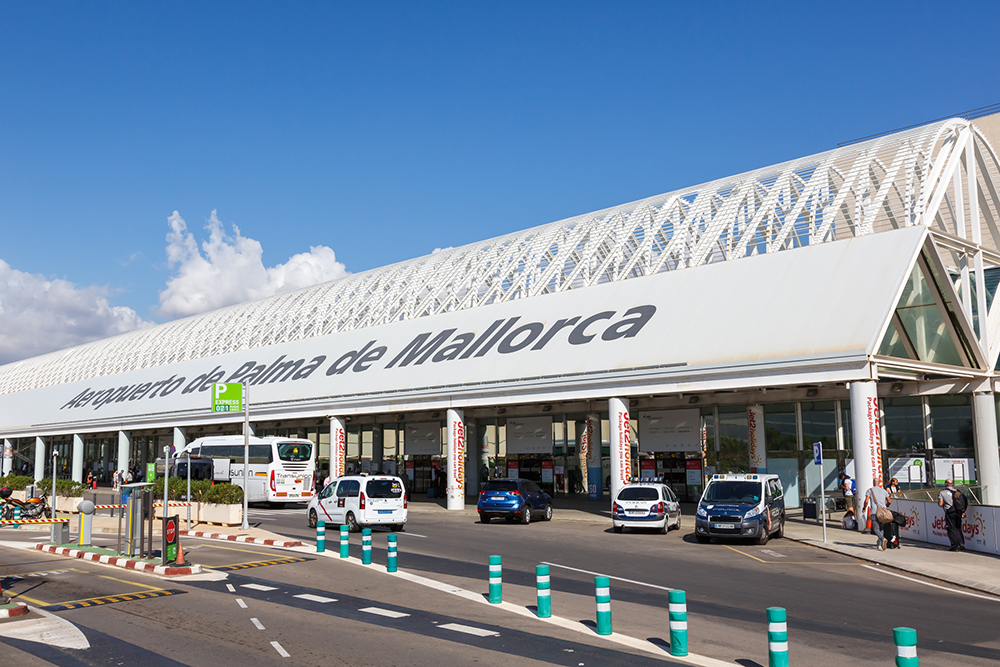
Air travel might be the fastest way to travel but the experience is frequently beset by landside problems, like crowded airport facilities, long queues, congested access roads, delays, and inconvenient parking.
The traditional approach to improving the customer experience was simply to expand airport facilities. However, it's much faster and more cost effective to make better use of existing resources simply by improving airport management; simulation analysis can make a significant contribution to better air travel by giving passengers more information, better service, and journey reliability. This is the focus of the Integrated Multimodal Airport Operations for Efficient Passenger Flow Management (IMHOTEP) research project, which is funded under SESAR 2020 Exploratory Research.
IMHOTEP’s vision is a multimodal European transport system, where different modes of travel are seamlessly integrated to give passengers a great door-to-gate and gate-to-door experience. The project team has created a concept of operations and a set of data analysis methods, predictive models and decision support tools that allow information sharing, common situational awareness and real-time collaborative decision-making between airports and ground transport stakeholders.
Modelling cross-modal interaction
The IMHOTEP team has developed a set of predictive tools to anticipate passenger flows. First, the passenger itinerary is predicted using big data, such as data from mobile phones, surveys, and flight schedules; second, passenger journeys are simulated using a model of the roads and public transportation that serve the airport terminal, and a terminal model for the passenger flow inside the terminal building.
The predicted passenger flows are simulated sequentially: the door-to-terminal journey is modelled using Aimsun mobility simulation solutions. The Aimsun model simulates all the movements between the passenger's home and the airport terminal entrance. Once inside the terminal, passenger movement from the terminal door all the way up to the boarding gate is modelled using CAST Terminal airport modelling software. This covers the whole door-to-gate process. After the plane lands, the process is then reversed to model the gate-to-door journey thus achieving full door-to-door modelling for air travel.
The IMHOTEP team applied these methodologies at Palma de Mallorca Airport (PMI) in Spain, and London City Airport (LCY) in the UK.
Agent-based modelling
At the heart of improving door-to-door travel is a method of modelling known as 'agent-based modelling'. Agent-based models represent each individual passenger, or group of passengers (like a family for instance), simulating how each 'agent' behaves and how they adapt to changing circumstances during their journey. In turn, each agent can be impacted by the actions of other agents. For example, heavy road congestion might cause many agents to switch from car to train, which will reduce traffic congestion and increase train crowding. Inside the airport terminal, similar problems can occur at boarding gates, passport control, security checks and so on. In short, this methodology enables us to simulate complex decision-making processes within complex systems.
In the IMHOTEP project, agent-based models help us compare different management strategies, which not only change the operating conditions but also customer behaviour. Imagine, for example, that the frequency of a certain public transport service is increased. This will not only reduce the waiting time of those who already planned to use it, but perhaps the shorter waiting time and availability of free seats will also attract agents who were perhaps originally intending to use some other mode of transport. Of course, agents who intended to travel by private car are less likely to switch to a different mode, but the key enabling functionality is the ability to accurately model the number of agents that will switch mode and then quantify its impact on the overall operations.
Or course, to ensure that any management action is better than doing nothing, when some disruption happens at least two sets of simulations need to be performed, the base case ('do nothing') and management actions ('do something'). And passengers react to the management action that has been taken.
Collaborative decision-making in real time
The IMHOTEP project envisions three interlinked management scenarios: delayed arriving flights; delayed departing flights; and a major motorway disruption (such as an accident) near the airport.
Each of these three scenarios would require some extra management to mitigate their negative impact on the functioning of the airport and, ultimately, the customer experience.
Imagine a delay scenario where several flights arrive later than expected during a peak season late afternoon at Palma de Mallorca Airport. Since the airport is likely to be operating near capacity, these late arrivals will stress the facilities, and result in high numbers of passengers crowding different areas. These passengers will need to reach their destination using one of the ground modes of transportation available, such as private car, rental car, taxi, Uber, bus, coach or airport shuttle. If the buses don't have sufficient capacity, how do the agents react? In the 'do nothing' scenario, some passengers might choose to wait, but others might take a taxi instead or hire an Uber. In the ‘do something’ scenario, we would know about these delays well in advance and increase the number of buses available at that time, thereby improving the customer experience.
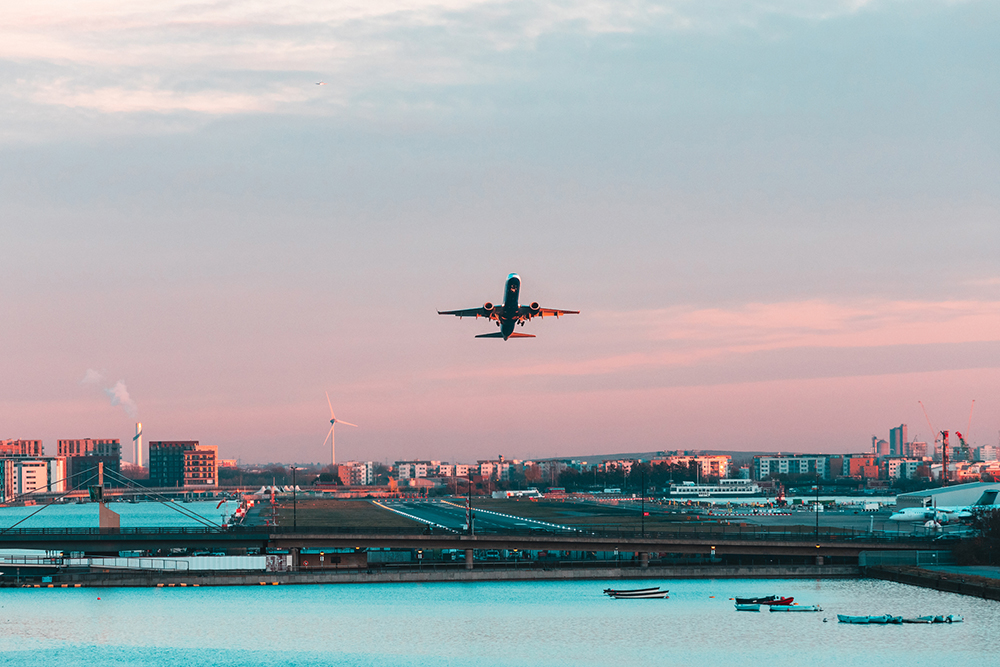
In our imagined scenario we've only considered the direct consequences when a plane arrives late, but of course this late arrival has a knock-on effect and will result in a series of follow-on late departures and arrivals, that will need to be managed.
Above, we have described the disruption qualitatively, but we need to extract some numbers from this description to rank and select the best management strategy. For a ranking of the best course of action to take, the agent-based simulation results yield key performance indicators (KPIs) for the terminal and the airport access and egress. With those KPIs is possible to rank the management strategies from best to worst according to the selected criterion.
Predicting, not reacting
You might wonder how exactly forecasting, modelling, simulating and quantifying passenger flows translate into seamless door-to-door journeys.
A key improvement is in the quality of advance information for passengers and stakeholders.
Using simulation, airports and airlines could give passengers the estimated departure time from their current location to reach the airport on time based on the current prevailing traffic and public transport conditions. This could be done through existing mobile phone apps.
Better knowledge of the current system status can also improve the decision-making process of the stakeholders. This might influence decisions about public transport operators (increase the frequency of service when needed), ground handling (improve resource allocation), terminal shops and restaurants (reallocate staff as needed), and even the airlines (delay a specific flight if necessary).
All these decisions could reduce the number of missed flights for passengers and improve the quality of service. This means that instead of reacting to any deviation from normal conditions after it happens, we can predict potential pinch points ahead of time and take proactive measures to reduce their impact. For instance, only open more security checks when the queue has reached a given length. If we know that queue will happen, we should be opening the additional checks just when the queue starts to grow. Thus, reducing waiting times, passengers with tight schedules make it to the gate on time, and others with more time have a more relaxed experience and can spend time at the airport’s shops and restaurants.
In summary, simulation analysis can clearly make a significant contribution to better air travel, giving passengers more information, better service, and journey reliability.
Content produced in association with Aimsun