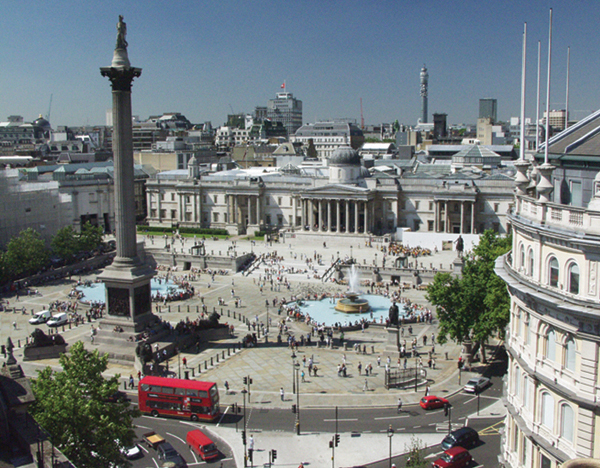
Data from the Journey Time Estimator is used in two ways: to generate about traffic conditions and recommended interventions; and to feed sources of personalised traffic information (Picture, TRL)
A new solution developed as a consequence of the UK's Freeflow project fuses GPS and UTC loop data to give more accurate predictions of journey times, benefting network managers and travellers alike. By Matt Cowley and Gareth Jones, Trakm8 and John Polak and Rajesh Krishnan, Imperial College London
Accurate real-time journey time information is a valuable tool both for network managers and in developing services for travellers. It is a key indicator of developing problems on a network and is also one of the few measures public users really understand.Travel time is already used on motorway and trunk road networks but has yet to fully penetrate into the urban networks typically controlled by Urban Traffic Control (UTC) systems. This is mainly due to the inherent variability in congested traffic, and also the challenges of actually collecting journey time data.
Measuring journey time
Typically, there are three ways to measure journey time on an urban network.Indirect measurement, using speeds from loop detectors in the UTC system, requires additional detectors and is limited due to the speed being measured directly only at a point.
Direct measurement, using GPS data or off-call cellular phone data, can be a measure of either point speed or journey time, depending on the technology used, but is generally a real-time measure of a single vehicle's progress through a network. While this works well on roads which carry higher volumes of traffic, sample sizes in urban areas with less traffic can be smaller and so data is often more sparse.
Direct measurement by Automatic Number Plate Recognition (ANPR) cameras gives journey time directly between fixed points but requires specific roadside infrastructure. Such systems also suffer from lag when the cameras are spaced far apart; a journey time cannot be measured until the vehicle being monitored leaves the link being monitored and in heavy congestion this may take some time. For many locations, direct measurement systems are used for monitoring performance and so the data is not available in real time.
Therefore, without additional infrastructure, no single source of journey time data works well in a real-time role.
Forecasting of journey times in the short term also has value, for example to assist satnav devices. Such devices need to know journey times for when a vehicle arrives at a link, not the present journey time at any given moment. Such prediction requires further tools which are often based on complex modelling and are difficult to deploy on a wide scale. Storing the data also provides a performance measure where ANPR is not available.
The Journey Time
EstimatorJTE provides a real-time short-term prediction of travel time for a vehicle just entering a link. It overcomes the low GPS sampling rate in urban areas by combining travel time observations from many different time periods to use existing UTC loop data. JTE does this both for both travel time estimation and also network state estimation, allowing congestion to be identified directly. The method generates not only a mean travel time but also other properties like short-term travel time variability.
The key innovation in the JTE approach, which is based on research undertaken at the Centre for Transport Studies, is to systematically learn and store the relationship between loop output and travel time previously recorded by vehicles with GPS devices. These relationships can then be used to determine the likely travel time for a given traffic state measured by UTC loops even if there is no GPS probe then present. The JTE prediction uses a pattern matching method called the k-Nearest Neighbour technique. When the output from loops for a new time period is presented, the method identifies the nearest travel time observations for when the stored loop outputs were nearest to the current loop data. The predicted travel time can be derived from this sample. In other words, the system answers the question, "What are journey times going to be like when traffic conditions are as they are now?" By looking into the way traffic conditions typically develop over time, it is able to add a prediction into approximately the next 15 minutes of how journey times typically develop.
Match point
The challenge is to achieve this match quickly enough to make a prediction valuable. Pattern-matching software can be used to find large quantities of data with a similar pattern to the current loop state but these require significant machine training, which is very time-consuming. The solution is to use a spatial pattern-matching algorithm developed by Trakm8 that will work out of the box using historic data. There is, of course, also much processing of data to look for errors, changes in road network and loop data trends, and so on.Trakm8 and Imperial College are now testing these predictions on a major test site using verification against video and ANPR data sources. Early results are encouraging, due to the ability to access very large amounts of GPS travel time data from Trakm8.
The Freeflow project
The core aim of the Freeflow project is to research how to deliver policy outcomes of improved safety, reduced congestion and safeguarding the environment, as well as measuring the changes made through demonstrations in the cities of London and York, and the town of Maidstone in the English county of Kent. The project partners are the University of York, Imperial College London, Loughborough University, the UK Department for Transport, Kent County Council,
alerts (which are compliant with the Urban Traffic Management and Control (
This twin use of data by both traffic managers through UTC and the travelling public is one of the basic principles of Freeflow. The project aims to fundamentally change how we use transport data, by using it to generate transport intelligence. Freeflow fuses transport policy requirements of better services with innovation from outside transport. This generates transport intelligence for urban areas to deliver services that users will really want to use and so improve how they travel. Trakm8's move from a pure fleet management approach into developing UTC products has been a major undertaking but the approach described here offers benefits for UTC systems around the globe. Moreover, as GPS devices proliferate the pool of relevant data will only improve over time.
Testing and validation
The JTE idea was in fact a by-product of Freeflow not foreseen in the original project proposals. It became clear that there was synergy between the structured data analysis approach of Imperial College and the availability of data from Trakm8.JTE is still in its test phase. It is about to go through a period of validation and verification in a range of typical UTC-controlled sites but by the middle of this year results will be published and the effectiveness of the tool demonstrated under a variety of typical urban conditions. If all goes as planned, Trakm8 will then be looking to roll out further deployments of the JTE as a fully UTMC-compliant plug-in module.
JTE is a good fit with the current economic climate. It provides a new intelligence source purely from processing existing data without adding roadside equipment and will help UTC managers to provide new services to road users and so better manage their networks.